Pathologywatch Implements AI Technology That Detects 5 Skin Lesion Types with Higher Sensitivity than Previously Published AI Model
Authors: James Requa, Tuatini Godard, Rajni Mandal, Bonnie Balzer, Darren Whittemore, Eva George, Frenalyn Barcelona, Chalette Lambert, Jonathan Lee, Allison Lambert, April Larson, Gregory Osmond
Highlights
- Including a large dataset of hand-drawn annotations increases the sensitivity of AI.
- Integrated supervised and semisupervised learning is superior to other AI models.
- Multilevel training permits subtyping, localization, and margin detection.
- Dermpath involvement in model development can ultimately improve model performance.
- Increased confidence in AI-assisted diagnoses can lead to improved patient care.
Abstract
Background
Skin cancer is the most common malignancy diagnosed worldwide, accounting for over 30% of all cancer diagnoses. Equally troublesome is the 77% increase in nonmelanoma skin cancers over the last several decades and a 250% increase in melanoma cases in children, adolescents, and young adults since 1973.
Objective
The goal of this study was to create an AI model that detects and classifies skin lesions with a higher degree of sensitivity than previously demonstrated. This AI model (i.e., Mihm) has the potential to match and eventually surpass expert pathologists and improve clinical workflows.
Methods
We produced an end-to-end multilevel skin detection system by combining supervised learning (SL) with semisupervised learning (SSL). This system detects five main types of skin lesions with high sensitivity and specificity. In addition, it detects subtypes while also localizing and providing margin status to evaluate the proximity of the lesion to nonepidermal margins.
Supervised Training Subset
- 2,188 random WSIs collected by PathologyWatch laboratory between 2013 and 2018
- Weekly supervised subset consisting of 5,161 WSIs from daily case specimens
Validation Set
- 250 curated daily case WSIs from PathologyWatch’s tissue archives
- Included 50 “mimickers”
Testing Set
- Composed of noncurated daily case specimens collected from PathologyWatch labs between July 20, 2021, and August 20, 2021
Results
By running the testing set through the Mihm Evaluation Pipeline, we could assess the performance characteristics of our AI model.
Our multilevel detection algorithm (patch-level, ROI-level, WSI-level) can subtype lesions with an AI overlay in the front-end digital display that localizes diagnostic ROIs and reports on margin status by detecting overlap between lesions and nonepidermal tissue margins.
Conclusion
This AI model, which is the result of collaboration among a team of dermatopathologists, detects five skin lesion types with higher sensitivity than previous AI models. It also provides end users with valuable insights in subtyping, localization, and margin status in a front-end digital display.
This end-to-end system can improve pathology workflows by doing the following:
- Increasing diagnostic accuracy
- Expediting the course of patient care
- Improving patient outcomes
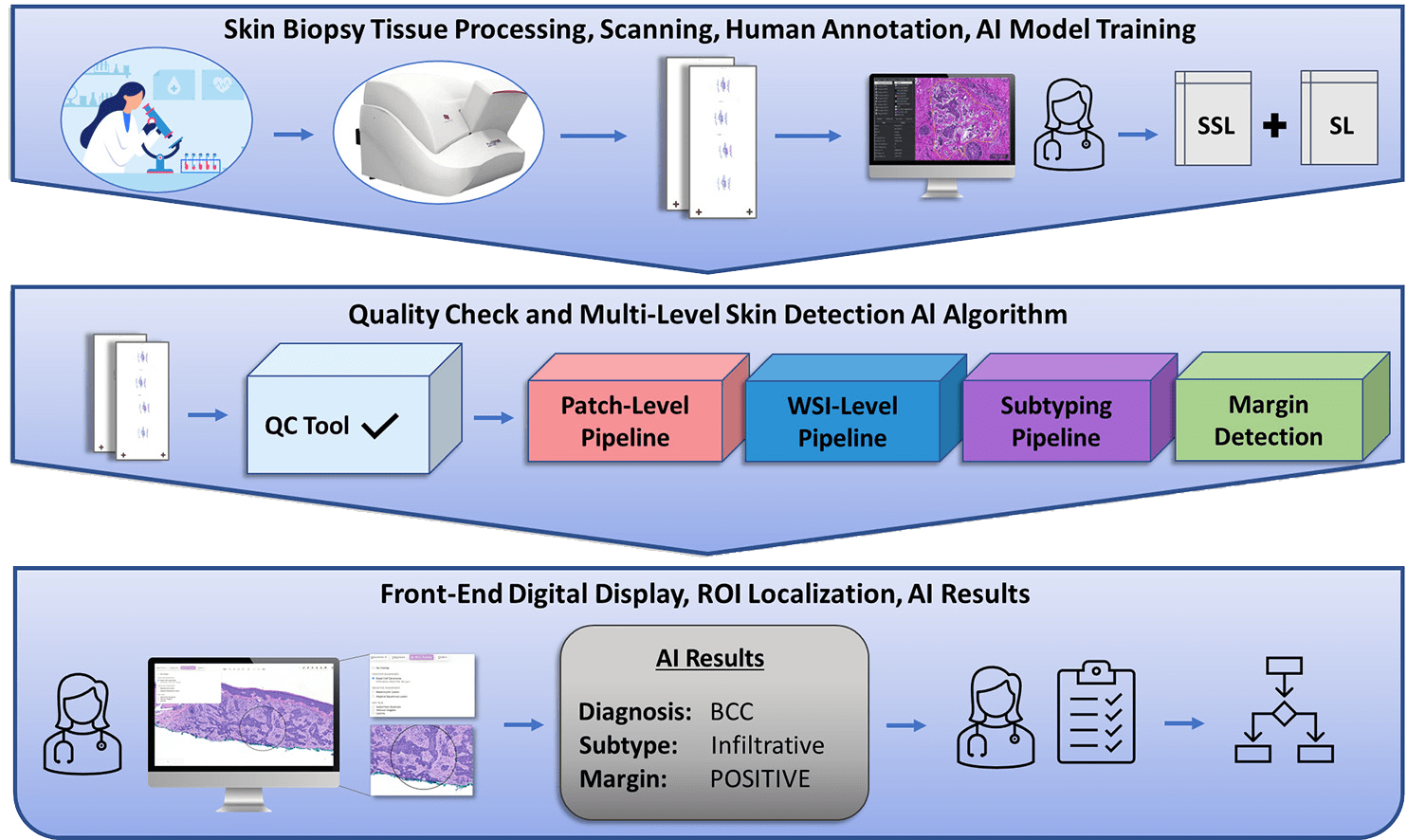